Be Data Literate Part III: Disaggregating Data To Identify the Root Cause of a Problem
Add bookmark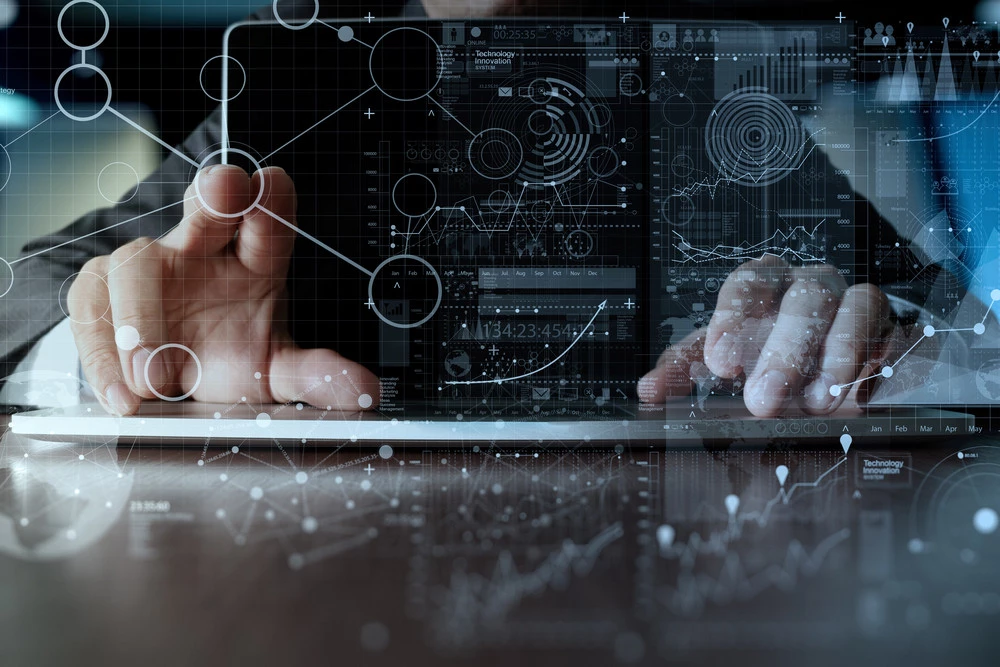
Editor's Note
Practically no problem in life–whether in government, business or elsewhere–ever presents itself as a case on which a decision can be taken.
There are many scientific approaches for finding the root cause of a problem. In this article, we present a simple (but not simplistic) statistical approach for "disaggregating" data to identify what statisticians sometimes call "an assignable cause.”
Executives have become computer literate. The younger ones, especially, are quite impressive with respect to their deep knowledge of the ins-and-outs of computers and related devices.
In many cases, they know how to get data. But many still have to learn how to "drill down" on that data in order to make it talk and tell the truth.
This same criticism can be levied at many journalists, talk show hosts, celebrities, politicians, government leaders and more.
Perhaps Will Rogers summed up the theme of this article when he quipped: "it ain't so much the things we don't know that gets us in trouble. It's the things we know that ain't so."
Descriptive Versus Prescriptive Statistics/Analytics
It's important to understand the difference between descriptive statistics and prescriptive statistics. Today's newer terms are descriptive analytics and prescriptive analytics. (Really old wine in new bottles).
Descriptive statistics refer to statistics that quantitatively describe or summarize a body of collected data or data set. Think percentages, arithmetic means, medians, standard deviations, ranges (high-low) and other measures of variation.
Prescriptive statistics involves the usage of statistical methodologies to help users find the root cause of a given problem and guide them toward a solution or remedy.
An Example
Descriptive measures don't suggest remedies to solve a given problem. Take, for instance, statistics on accidents: They tell you about the number of accidents in the workplace, in the home and on the road.
Indeed, one can even determine if there is a trend in the frequency of accidents using data collected on accidents over time.
But this statistic can't, nor does it pretend to, tell you how to reduce the number of accidents.
A statistical-based performance measurement analysis methodology, if properly used, can locate the root causes of, say, the majority of accidents and point the way to taking corrective action in reducing their frequency.
This “descriptive statistic” has its uses. you would be terribly irresponsible and totally misguided if you thought a descriptive statistic provides a prescriptive answer.
In short, what can be done to lower the accident rate? There is a world of difference between “knowing” a problem exists and “doing” something about it.
A Prescriptive Methodology: Finding The Root Cause of a Problem Via Continuous Subgrouping/Disaggregating Your Data
Someone tells you, "our invoice error rate equals 10 percent." You shake your head and mumble something to the effect of, "That seems rather high. Do we know why it's so high?"
The basic question is whether the aggregate number or percentage (the result for the total group) conceals differences among subgroups. For example, can the aggregate statistic,10 percent incorrect invoices—be subdivided into sub-groups?
Definitely. One basis for subdivision is the two shifts doing the invoicing. For example:
Table One
Shift | Invoice Error Rate | Number of Invoices Processed |
8 a.m.-4 p.m. | 0% | 1,000 |
4 p.m.-12 a.m. | 20% | 1,000 |
2,000 |
Aha! This subgrouping reveals a significant difference in invoice error rates by shift. All incorrect Invoices were made during the second shift. At first glance, they seem to be the chief culprit.
Since the number of invoices processed is equal, it's unnecessary to use a weighted average to arrive at the average invoice processing error rate.
In this case, we add the invoice error rates and divide by the total number of rates (0 percent + 20 percent /2 = 10 percent). But, as we now see, the second shift appears responsible for all the invoice errors.
This Process Can Be Extended to Several Additional Subdivisions
By introducing additional subdivisions, and finding significant differences or variations among subgroups, we can move closer to determining the “root cause” of the incorrect invoices.
For example, the experience level of the employees working the two shifts supplies a possible explanation for the excessive number of the incorrect invoices.
In this organization it was discovered that all new workers were routinely assigned to the second shift. Our chief focus is now on the night shift.
So, we now subdivide the night shift workers into two distinct subgroups–namely, experienced people and inexperienced people.
Table Two
Experience Level | Invoice Error Rate | Number of Invoices |
Experienced People | 0% | 500 |
Inexperienced People | 40% | 500 |
1,000 |
Table Two indicates that new workers (i.e., inexperienced people) were responsible for all the incorrect invoices(200/2000), if the subdivision process stopped at this point.
It would appear that more intensive training of new billing staff could dramatically reduce the rate of incorrect invoices. Sure looks like the right remedy–doesn't it?'
However, if we introduce one additional basis for subdivision, we learn more about the “root cause” of Incorrect invoices.
What is the composition of the new invoice people on the second shift? That is, can the subgroup be further subdivided on the basis of some other characteristic yet not under investigation?
Table Three indicates that inexperienced (i.e., night shift) billing staff are of two kinds: (1) full-time employees and; (2) part-time temporary staff.
Table Three
Inexperience People | Invoice Error Rate | Number of Invoices |
Full-time Employees | 0% | 250 |
Part-time Employees | 80% | 250 |
500 |
Table Three reveals that part-time temporaries are the cause of all the incorrect invoices (200/2000). Why? The full-time staff received thorough training in the invoicing policies and procedures.
But—and this is a very big "but"—the part-timers are brought in during peak shipping periods and are often thrown at the problem, receiving no training.
That this happens shouldn't surprise you. However, it often gets overlooked when confronted with aggregated numbers.
People see what is presented to them; what is not presented tends to be disregarded.
And what is presented, more often than not, are "problems couched in aggregate data"—especially in the areas where performance falls below expectations—which means that managers tend not to see the real cause of the problem.
The Root Cause, Once identified, Can Be Eliminated via Management Action
How can the number of incorrect invoices be eliminated? Less dependence on part-timers in critical areas, and more effective training of the temporaries that are used.
This particular organization stopped hiring temporaries for billing and invoice costs dropped eighty percent.
Management must change its policies. In this case, the hiring of ill-trained office temporaries was eliminated. Management took an action to remedy the problem. The right action!
There was a corresponding savings in the customer support area. Customers no longer had to deal with chronically incorrect bills. This example is a bit oversimplified but it is based on an actual case.
Jim Harrington, a Deming missionary who worked at IBM for 35 years, estimates that 50 percent of the costs of every billing system are attributable to “screw ups." Why continue to pay that money?
To Reiterate
- An aggregated performance measurement is of limited diagnostic value.
- Through the process of isolating and analyzing variation among relevant subgroups, you can locate the “root cause” of the problem.
- Management action is required to deal with the “root cause” of the problem. (A reminder: A decision is not an action. A decision is a good intention. Decisions must be converted into action).
- Faulty conclusions and/or policies inevitably flow from a data-set that is not homogeneous with respect to the performance measurement under investigation. In other words, the wrong problem is being solved.
- Statistical procedures detect significant variation among subgroups. If significant differences in a performance characteristic (because of thoughtful subdivision of a data set) are found to exist, the reasons for the variation must be investigated been eliminated from the process.
- After the “causes” of the variation are discovered and eliminated, the performance measurement under investigation improves.
Afterword: Requiring Statistics 101 For Purveyors and Receivers Of Information?
When it comes to a misunderstanding of what the data actually says, or fails to say, politicians of all stripes and various media outlets are second to none.
For example, the wage gap myth which we've heard so much about these last few years, ignores many common sense variables and subgrouping that render most wage comparisons meaningless.
Still another example relates to the false narratives about "intentional discrimination" by police forces nationwide. (Heather Mac Donald's runaway bestseller entitled War On Cops, provides many examples of the pitfalls of aggregated data).
But the wage gap myth and faulty statistical analyses fueling civil unrest are just tips of the iceberg!
Not a day goes by that we are not being subjected to cheating charts, meaningless statistics, improper comparisons, and erroneous conclusions.
Worse, by failing to apply what might be called elementary statistical analysis to a variety of societal and management problems, it's near-impossible to separate a problem's symptoms from its causes.
To arrive at the definition of the real problem and the development of alternative solutions that could successfully solve the problem requires an approach thoroughly grounded in scientific and statistical thinking.
From this point forward, we ask you to internalize this basic truth: Overly-aggregated data misleads, misinforms and misguides.
In today's age of fast history, we believe, all responsible people should acquaint themselves with the practical aspects of statistical analysis (e.g., spotting far-fetched estimates, the dangers of neglecting important omissions in data, understanding the significance of the all-important definition).
More than 130 years ago H.G. Wells said: “Statistical thinking will one day be as necessary for efficient citizenship as the ability to read and write.”
Missed Part I and Part II? No worries!
See what disaggregated data is, and why it’s important for management decision-making in Part I.
Then check out several sub-grouping examples that provide you with a deeper understanding of the dangers of statistical ignorance with respect to failure to analyze presented metrics or data at the appropriate level of aggregation in Part II.