Straight Talk For Twisted Numbers: Perils of Polling Part I
Add bookmark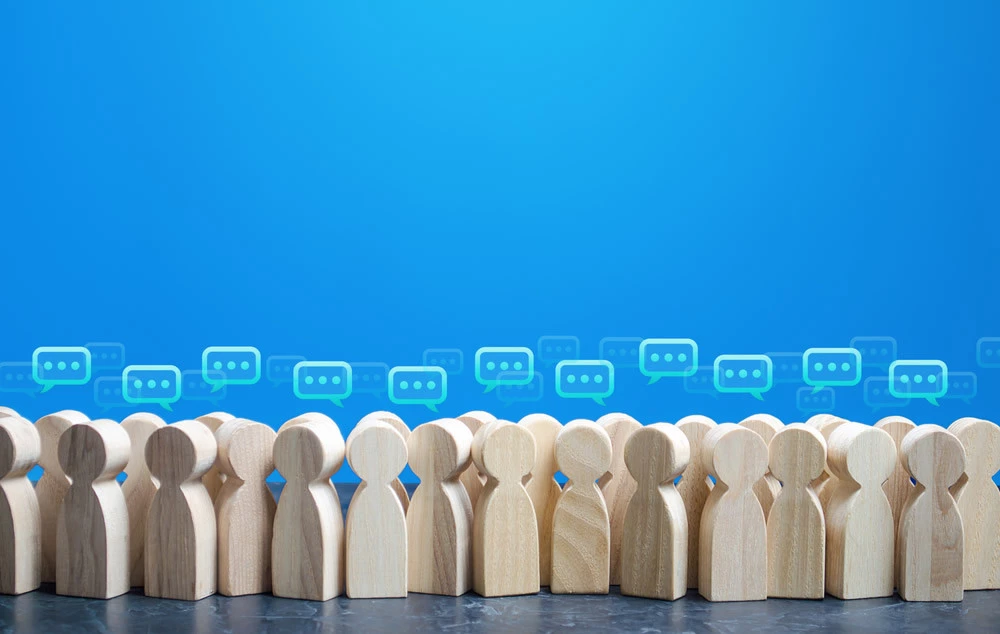
Editor's Note
The field of data science is wide in scope. Data science is a relatively new term.
But, properly viewed, data scientists are really yesterday's MS/Ph.D. level statisticians.
A famed Princeton statistician named John Tukey decided in the early 70s he much preferred to be called a data scientist rather than a statistician.
And so, according to many quantitative professionals, the field of data science was born.
Statistical analysis for decision-making, for nearly 100 years, was divided into three distinct groupings–namely:
- descriptive statistics
- prescriptive statistics and
- predictive statistics
Of course, today's data scientists use the same three groupings to define the field of data science.
But with one clever twist–they took out the word “statistics” from the above statistical decision-making categories and substituted the word analytics” (i.e., descriptive analytics, prescriptive analytics, predictive analytics).
And now that we've gotten this out of the way, let's tell you the purpose of this article and our planned series of articles to help L&D and other executives better understand the rapidly growing field of data science which includes AI (all AI is based on predictive analytics/business econometric model building).
Don't let this scare you! We think you'll find it very easy to understand and very useful with respect to simplifying seemingly complex problems.
The purpose of this article is to explain, in relatively simple terms, one aspect of the decision sciences executives are frequently concerned/involved with. That is: either interpreting or actually carrying out a sample survey or poll.
Indeed, many organizations are constantly “sampling” customers to determine the viability of a potential new product offering or a new pricing strategy, how to improve the total customer experience and dozens of other areas related to creating, keeping and growing customers.
Part I provides important background information that will help readers ask better, more informed questions related to survey results.
Part II will laterally extend some of the subject material in Part I and make it more relevant in terms of business examples.
Introduction
This is a story with a lesson. The University of Pennsylvania Wharton School's Dr. Morris Hamburg in a much-acclaimed breakthrough textbook Statistical Analysis for Decision-Making used this unforgettable example to illustrate different interpretations to the same set of facts or statistical data.
"The heroine of our story… Is a grammar school teacher, who wanted to demonstrate the harmful effects of drinking liquor to a class of eight-year-olds. She placed two glass jars of worms on her desk…
...Into the first jar, she poured some water. The worms continued to move about, and did not appear to have been adversely affected at all by the contact with the water…
Then she poured a bottle of whiskey into the second jar. The worms became still and appeared to have been mortally stricken...
The teacher then called on a student and asked, 'Johnny, what is the lesson to be learned from this experiment?'...
… Johnny, looking very thoughtful replied,' I guess it proves it's good to drink whiskey because it will kill any worms you may have in your body'…"
Lesson Learned?
Statisticians would call this a prime example of a statistical non sequitur, a Latin term meaning "it doesn't follow."
Dr. Hamburg's point? Even when statistical data are above reproach, much leeway still exists for individuals to interpret them in a manner that best supports their mindset, level of subject knowledge and/or prejudices.
Stanley Schor, a noted bio-statistician, once colorfully described this as: "Statistical results are like expert witnesses; you can get them to testify for either side."
Little Johnny was well-meaning. His interpretation of the outcome of the whiskey experiment was based on the mindset and/or the perception (i.e., knowledge and experience) of an eight-year-old.
Take-Away Message
We all can forgive little Johnny.
But it's becoming more difficult to forgive those that leap from a statistical fact to a convenient conclusion – without the conclusion jumper being handicapped by any consideration of alternative conclusions, required knowledge of the subject matter or even by any rules of simple logic.
A timeless verse from Robert Browning best describes the painful truth about fixed, rigid mindsets:
"As in your sort of mind, So in your sort of search; you'll find What you desire."
Will Rogers also addressed the issue of fixed mindsets when he said: "It ain't so much the things we don't know that gets us in trouble. It's the things we know that ain't so…"
Why People Can View the Same Facts Differently: The Role of Perception
Perception consists of two components – namely, knowledge and experience. People with different knowledges and experiences can view the same facts differently.
What A sees so vividly, B does not see it all. And, therefore, what A argues has no pertinence to B's concerns and vice versa.
"Just as the human ear," Peter F. Drucker wrote, "does not hear sounds above a certain pitch, so does human perception altogether not perceive what is beyond its range of perception."
In his much-celebrated book Innovation and Entrepreneurship, Peter F. Drucker reminds us that "When a change in perception takes place, the facts do not change. Their meaning does."
How we see things influences how we understand them and how we respond to them.
To repeat: Knowledge and experience determine our perception (or mindset), that is, what we see and how we see it.
Mark Twain once quipped: “When I was a boy of 14, my father was so ignorant I could hardly stand to have the old man around. But when I got to be 21, I was astonished at how much the old man had learned in seven years.”
Perhaps when 8-year-old Johnny is 18, his response/interpretation to the same facts will change. We certainly hope so.
Questioning Data Collection Methods: An Example You'll Likely Never Forget
There are three aspects of all sample survey/polling projects. These are data collection, analysis of the collected data and the presentation of the analysis.
Relatively few people question the data collection process or methodology. The following example puts a spotlight on how flawed data renders any conclusions from a survey/poll useless.
For years, in the UK, it was reported instigators of barroom brawls were more likely to be killed in such fights than those defending themselves.
Sociologists, psychologists and substance abuse agencies studying these phenomena formulated theories, wrote scientific-sounding white papers/articles and offered lucrative speaking engagements to explain this rather unexpected outcome.
Many of these explanations used "highfalutin" psychological/behavior modification terms that sounded very learned and were in many instances very convincing.
The Royal Statistical Society (RSS) – a very prestigious data science organization - however, was somewhat suspicious of these results and set out to check for themselves their accuracy and reliability.
The RSS's Ph.D. level statisticians eventually discovered the data were indeed questionable as the collection methods did not encourage truthful information.
What Did The RSS Discover?
What the statisticians discovered was every time a barroom brawl ended in a fatality or with serious injuries, the investigating police officer dispatched to investigate the incident would invariably ask: "Who started this?"
Witnesses would immediately point to the hapless victim lying on the floor and say "he did it."
The response was duly noted and because of the victim's inability to dispute the accusation, the witnesses' assertions were usually taken to be true.
The data used for analysis was derived from police officer reports. Perhaps the phrase "garbage in/garbage out" is very applicable to many analyses based on questionable data collection methods.
Lesson learned? When studying/reviewing a statistical analysis or the results of polls, ask yourself and relevant others: "How was the data collected?"
A Neglected Truth About Opinion Polls
Neil Postman in a marvelous book entitled Technopoly delightfully exposed an inherent weakness in interpreting survey results:
"Pollsters ask questions that will elicit yes or no answers. Polling ignores what people know about the subjects they are queried on.
In a culture not obsessed with measuring and ranking things, this omission would probably be regarded as bizarre.
But let us imagine what we would think of opinion polls if the questions came in pairs, indicating what people 'believe' and what they 'know' about the subject.
If I may make up some figures, let us suppose we read the following:
The latest poll indicates that 72 percent of the American public believes we should withdraw economic aid from Nicaragua.
Of those who expressed this opinion, 28 percent thought Nicaragua was in Central Asia, 18 percent thought it was an island near New Zealand... and 27.4 percent believed that ‘Africans should help themselves,' obviously confusing Nicaragua with Nigeria.
Moreover, of those polled, 70 percent did not know that Americans give economic aid to Nicaragua, and 23 percent did not know what ‘economic aid’ means.
Were pollsters inclined to provide such information, the prestige and power of polling would be considerably reduced."
A Point to Ponder – A Useful Digression
Many surveys/polls of representative groups of Americans revealed a significant percentage of the population very worried about the future of America. And with good reason!
Many of these polls ask questions relating to the ever-worsening border invasion… crime out of control in major cities… prices – especially food and energy – going up at alarming rates… America going from exporter to importer of fossil fuels… mobilized efforts to defund the police, encourage the demoralization of Immigration & Customs Enforcement (ICE) officers driving them to resign… and so much more!
What's happening is increasingly being covered in publications such as the Washington Post, New York Times, CNBC, CNN, Fox News and a host of other liberal/conservative media companies.
One always wonders how much people really know about what's happening with respect to the government creating massive new dependencies… giant increases and welfare and entitlement payments coupled with no work requirements and no education training requirements for receiving what could amount to both cash and non-cash incomes of $60-$65,000 per year… the predicted future shrinkage in value (if things continue the way they're going) of pension funds and 401(k)s and more.
Most importantly, what do people really know about how today's current events will specifically impact their safety, future income, freedoms and all the rest?
Drucker in his book Managing in a Time of Great Change (Chapter 25) (spelled out in detail why the welfare underclass (which America is now expanding at an alarming rate) threatens middle-class life, middle-class culture, middle-class values.
Without doubt, observed Drucker, “Modern welfare destroys… It does not build competence… It does not alleviate poverty (even though it provides middle-class or near-middle-class incomes to many welfare recipients).”
We suggest you re-read Neil Postman's comments above again. It will help you better understand the varying results of polls with respect to the topic of "Is America In Decline?"
Hopefully, you'll now be more questioning with respect to the way data is collected, analyzed and presented.
How to Guarantee Wrong Conclusions From Polls or Surveys – A More In-Depth View
Let's get right to it. Classically trained statisticians call it the statistical universe or population.
What is a statistical universe? The statistical universe consists of all things about which conclusions are to be drawn.
For example, in a study of price fluctuations in the price of heating oil in New York City from 2016 to 2021, the statistical universe would include every price change which had occurred during the specified time interval.
If the scope of the study were expanded to cover a larger territory or a longer period of time, the statistical universe is correspondingly enlarged.
Obviously, the term statistical universe is an elastic one and varies in its precise connotation with every statistical undertaking.
In general, the statistical universe may be defined as a totality embracing every item which might have been brought under observation had a complete enumeration been effected.
The Need for Sampling
Lack of time and money renders it impossible to make a complete survey of most statistical universes. Thankfully, it's not necessary to survey the entire statistical universe.
Why? Because hard-working, brilliant statisticians discovered how to get the same information from carefully selected, relatively small samples.
Making the correct inferences on small-size samples taken from large or sometimes infinite statistical universes is the subject matter of basic and advanced statistics.
If the sampling process is properly carried out, an analysis of the samples makes it possible to infer information about the statistical universe within the limits of unavoidable chance errors of sampling – the so-called margin of error.
Unfortunately, many courses in basic statistics fail to emphasize one critical point, namely, if the statistical universe is improperly defined, the powerful techniques of inferential statistics (making inferences on the basis of samples) are of little or no value.
The Classic Example of a Poorly Defined Statistical Universe
Undoubtedly the most widely publicized illustration of a poorly defined statistical universe is the one concerning the Literary Digest's error in predicting the winner of the presidential election of 1936.
Indeed, this is the example most often cited by W. Edwards Deming.
During the 1936 election campaign between Franklin D. Roosevelt and Alfred M. Landon, the Literary Digest magazine sent mock ballots to a large list of people whose names appeared in telephone directories and automobile registration records. (Their lists also included their own magazine subscribers, country club members, and the like).
Over 10 million mock ballots were sent out; 2.4 million ballots were returned by respondents; 7.6 million were not returned.
On the basis of 2.4 million returned ballots, the Digest predicted Landon would win by a comfortable margin––indeed, a landslide.
As it turned out, however, Roosevelt received 61% of the votes cast, a proportion representing one of the largest majorities in American presidential history.
How Could They Be So Wrong?
Polls only represent the people who are in a statistical universe and who respond to them.
Despite the sample's huge size, this election became a textbook case of a biased sample: all of the sample's component groups (or sub-groups) were heavily Republican.
Let's get more specific. There were two important reasons for the erroneous prediction--namely: (1) an incorrectly defined statistical universe and; (2) non-response bias.
Everyone with telephones and automobiles, in 1936, was in a higher economic group than those people without these two “luxuries.”
The point? There was a bias inherent in the statistical universe.
A large percentage of the voting population would not show up in telephone directories, automobile registrations, and club memberships.
The statistical universe was improperly defined––it tended to be biased in favor of higher-income groups. Higher-income groups (in that time period) tended to be Republican.
In the 1936 election, there was a strong relationship between income and party preference. Lower-income groups tended to be Democratic.
Bias in the Sample Selection Process
Classically trained statisticians define a bias as a persistent error in one direction. What does this mean?
No matter who you sampled from the Literary Digest's statistical universe, there was a high probability a relatively affluent person would be selected.
To repeat: the statistical universe selected was slanted towards middle and upper-class voters… and excluded most lower-income voters. And, in reality, there were a great many low-income voters in 1936.
Nine million people were unemployed in 1936.
Said Deming: "With regard to economic status, the Literary Digest poll was far from being a representative cross-section of the population. Then as now, voters are generally known to vote with their pocketbooks.”
It should be mentioned – indeed, emphasized – George Gallup was able to predict a victory for Roosevelt using a much smaller sample of about 50,000 people.
Gallup's statistical universe consisted of a representative cross-section of the population.
The Literary Digest poll sample size was 2.4 million people. This illustrates a poorly defined statistical universe cannot be cured by increasing the size of the sample, which in fact just compounds the mistakes.
Non-Response Bias
The second problem with the Literary Digest poll was that out of the 10 million people whose names were on the original mailing list, only 2.4 million responded to the survey.
It was then a fact that individuals of higher education and higher economic status were more likely to respond to mail questionnaires than those of lower economic and educational status.
Therefore, the non-response group - 7.6 million people - contained a high percentage of the lower economic status group.
The 2.4 million people who responded to the questionnaire tended to be from a higher educational and economic status group.
A case study involving the Roosevelt/Landon poll from the University of Pennsylvania's Wharton school describes the situation as follows:
“When the response rate is low (as it was in this case, 24%), a survey is said to suffer from non-response bias.
This is a special type of selection bias where reluctant and non-responsive people are excluded from the sample.”
Take-Home Message
It's a major error to take a sample from the statistical universe which differs considerably from the “ideal” statistical universe about which you want to draw valid conclusions.
Considerable time must be spent in defining the ideal statistical universe and every attempt must be made to draw a representative sample from that universe.
Every day we hear about making, say, pricing decisions in today's inflationary economy (to keep existing customers) based on sample information derived from polling existing customers.
Important point: The statistical universe in many of these cases should also include non-customers, that is, people who do not currently buy the company's products/services even though they should be (or might be) in the market and responsive to price changes and changes in the customer-getting value proposition. (See Part II of this article).
Always be on the lookout for a poorly defined statistical universe.
Fancy calculations relating to sampling errors, interval estimates, and statistical tests are meaningless if the statistical universe from which conclusions are drawn is incorrect.
Finally, beware of non-response bias. Said the Wharton school case study: “People who respond to surveys are different from people who don't, not only in the obvious way (their attitude toward surveys) but also in more subtle and significant ways."
Summary & Conclusions
Of late, we constantly hear or read that "You can't argue with science."
However, in many instances, those making that statement are confusing what might be called the hard sciences (e.g., physics, mathematics, virology, biochemistry) with data science.
Unfortunately, it seems increasingly clear many are data science illiterate. Further, what sometimes gets presented as data science can best be described as "data science fiction."
Stay tuned to other articles in this series that will discuss many aspects of prescriptive and predictive analytics.
Hopefully, this will enable executives to better communicate with data science professionals… and with relevant others about the results of surveys and polls.